Artificial intelligence in the age of adisciplinarity
Enkelejda Miho puts different fields to work to develop new medicines, diagnostics, and a better understanding of the health-disease continuum
Prof. Dr. Miho, there are many ways to apply AI to research in life sciences. What is your team atthe FHNW School of Life Sciences focusing on?
One of our applied projects for therapeutics is the design of broadly neutralizing antibodies against the Dengue virus. Currently, there is no medicine to treat the virus – existing therapies focus on alleviating symptoms, while current vaccines only protect against some serotypes.
To design an antibody-based treatment, we investigated the B-cell immune response to Dengue virus antigens in mice and humans. Specifically, we looked at B-cells in murine bone marrow, which is where long-term immune memory is headquartered. Using high-throughput single-cell sequencing, we obtained 47.8 million raw reads of B-cells and the antibody sequences they produced after exposure to Dengue antigens of various complexity.
Then we had to make sense of this large-scale data. We applied machine learning to detect patterns in the antibody repertoires and sequences against Dengue. But do these findings apply to humans? To determine this, we looked at memory B-cells (MBC) from human patients affected by acute Dengue Virus 2.
We concluded that, in both mice and humans, exposure to Dengue generates long CDR3s and Y-rich motifs in the antibody repertoire. Our hypothesis is that these features could be responsible for quickly activating memory B-cells and generating broadly neutralizing antibodies that bind to the E protein on the surface of Dengue viruses.
Not only did we identify promising antibody sequences for neutralisation of infections but we also gained new knowledge about the antigens. Dengue whole E-2 complex antigen generates the most diverse antibody response and could therefore be suitable for the development of new vaccines against the virus.
The next step of this project will involve humanizing antibody sequences we isolated in mice so that they can be used as potential therapeutics for Dengue infection and to prevent severe disease.
What about Dr. AI? Do you see a big role for AI-assisted diagnosis?
I see an increasing potential in using AI to support precise diagnosis in diseases that can be difficult to identify because of their overlapping and unspecific symptoms, for example autoimmune and rare diseases. This involves unravelling which data is most useful for diagnosing which diseases, and finding ways of combining and standardizing that data. My team is integrating clinical data from electronic health records, laboratory values and multi-omics data. We have developed new integration methods that can do this.
The second element involves benchmarking machine learning models to determine how well a given model can identify disease and which model is the most accurate. We have enhanced our findings from real patients with virtual patients – like a digital twin of a patient. We have worked with European hospitals and medical experts from Germany and France, and we hope to apply the findings to implement digital clinical trials to improve not only diagnoses but also therapy regimen outcomes.
To bring software as a diagnostic device to hospitals and the market, we maintain close ties with Swissmedic and the Swiss Network for Digital Medical Regulation.
In both examples you’ve given, it seems that understanding the immune system’s response to disease is key. Which research directions will you take in the future?
Often, the immune system reveals what is going on before disease strikes. We’d like to map out the immune imprint of diseases – a colossal task. I’m interested in exploring and revealing the relationships between infection, cancer and immune diseases.
To do this, my team is studying molecular dynamics. These are giant networks that feature large input data, which AI is well suited to handle, but also complex combinatorial intermediate scenarios, for which quantum concepts and quantum computing could help. I expect we will use a hybrid approach to uncover the secrets of how infection, cancer and immune diseases are related.
AI and life sciences are coming together. Do you consider yourself an interdisciplinary researcher?
I think it’s useful to use the concept of adisciplinarity instead of interdisciplinarity. Multiple disciplines are evolving in parallel. Some fields are merging, while others are splitting apart (e.g. the emerging biochemistry between biology and chemistry). My team and my students use concepts from life sciences, computer science and business to solve a problem at hand, by applying principles from various and emerging disciplines. So we are agnostic to disciplines in a sense.
This applies not only to research projects but also to teaching, for example in the Digital Transformation in Healthcare course and even in the HackLife hackathon we’ve been organizing with Prof. Javerzac at the FHNW School of Life Sciences since 2022. We ask organisations to present their industry-relevant challenges, and we use a variety of approaches including AI, ML and quantum computing to develop solutions that should be practical to implement in a business context.
And a final word about ‘hacking’ – it used to mean learning to code, now it means learning to prompt. Large language models have changed the rules of the game. The most famous is ChatGPT and there are many others. Using language to formulate prompts is the latest development that might revolutionise not only complex scientific disciplines but everyday work and life.
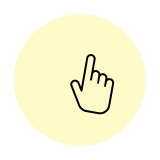
Key facts | |
---|---|
Partners:
| University Hospitals Strasburg, Mainz, Freiburg; Universities of Pavia, Pamplona, Utrecht, Heidelberg; Institute Pasteur, University of Oslo |
Financing: | SNSF EUREKA 32ER30-213721, Wellcome Trust Innovator’s Award |