Enhancing Third Party Patent Monitoring with Machine Learning and Natural Language Processing
Application of state-of-the-art NLP models increases efficiency of third party patent monitoring in the nutrition and bioscience industry.
Objectives
Identifying relevant third-party patents using transformer-based classification models.
Background
Every year millions of patents are being published worldwide covering a vast variety of topics. Patent applications generally average ~10,000 words using unique, highly context dependent, meticulously wordsmithed language (aka “legalese” or “attornish”). Monitoring third party patents is a crucial element of business development and innovation for many companies.
Keyword-based search strategies can help to reduce screening efforts by subject matter experts (SMEs). However, even with a highly customized framework of rules it is challenging to make a selection containing mainly relevant patents. This results in a substantial time investment to manually screen irrelevant patent documents.
Results
The Institute of Data Science FHNW successfully developed a transformer-based classification model ensemble trained on third party patents annotated by DSM SMEs. A field study revealed that this model allows more efficient patent screening reducing substantially labor costs. Moreover, the model allows the pool of patents screened for relevance to be expanded, hence enabling identification of additional potentially relevant patents. Based on the PoC success, DSM intends to implement the solution on premise as a next step.
Information
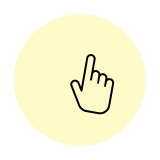
Client | |
Execution | |
Duration | 6 months |
Team | Prof. Dr. Daniel Perruchoud, Dr. Fernando Benites, Dominik Frefel, Joshua Meier |